- Blog
- Scams pose a threat to the gig economy apps
Scams pose a threat to the gig economy apps
Learn how to fight back in the latest episode of Trust & Safety Mavericks
Subscribe to the Incognia Newsletter
Even with economic tides turning, one thing seems certain—the gig economy is here to stay. From rideshare apps to food and grocery delivery to small tasks like building Ikea furniture, these small jobs or “gigs” have helped people supplement or even replace their income. Most gig workers today access jobs and other resources via marketplace apps that connect them with customers and support teams, such as Uber, Lyft, or Instacart.
These apps are excellent for connecting independent contractors with local people who want their services, but unfortunately, fraudsters and other bad actors are just as capable of downloading these apps as anyone else. Scams affect the gig economy as much as any other sector, but, fortunately, gig apps also have resilient anti-fraud tools at their disposal to help get rid of bad actors.
Key TakeAways
- Fraud and policy abuse are related, but not completely the same—while fraud might involve criminal actions like account takeover and subsequent theft, policy abuse is about using the app counter to the creators’ intentions for personal gain
- Scams on gig economy apps can be initiated by workers against consumers, consumers against workers, or from either user group against the marketplace itself
- Precise, spoof-resistant location technology can help by making fraud operations unscalable and thus more expensive for bad actors
Andre Ferraz, CEO and co-founder of Incognia, and Vishal Kapoor, Director of Product at Shipt, recorded a webinar about gig economy scams hosted by About-Fraud’s Ronald Präetsch. In this webinar, also available as episode four of the Trust & Safety Mavericks podcast, they discussed the types of scams prevalent on gig economy apps, the difference between fraud and policy abuse, and what platforms can do to keep their workers and customers safe from bad actors.
Challenges facing the gig economy: Fraud vs policy violations
Though the workers who use gig economy apps are usually independent contractors, they still have to abide by the policies set forth by the app. The app is often the intermediary, facilitating payments and communication between the customer and the gig worker, meaning that workers and customers alike are beholden to the community guidelines or terms of use as laid out by the apps trust and safety experts if they want to transact smoothly.
Sometimes workers or customers violate these rules without breaking the law. What they’re doing may not technically be fraud, but it still might cost the company money or damage the experience for other customers. This is what’s called “policy abuse” or “policy violation,” and while it’s different from outright fraud, there’s overlap in both the damage it causes and the methods used to perpetrate it.
While policy abuse and fraud both have the potential to negatively impact the user experience and cause financial damages to the company, fraud is typically more criminal in nature. For example, account takeover and the subsequent theft of funds is a common type of fraud challenging gig economy and other apps that enable users to hold cash in their accounts.
Gig economy scams can flow in all directions
Gig economy apps are all about making connections–namely, connecting customers with workers, and customers and workers with the app itself. One unique aspect of the trust and safety challenges facing gig economy apps is that fraud can be perpetrated by multiple different stakeholders. As Ferraz explains:
"Now the combination of attacks can happen in several ways. You have cases of consumers scamming the couriers, couriers scamming the consumers, fake listings, and fake restaurants or stores. You have all of these types of things going on making it more complex."
Consumers might scam gig workers, gig workers might scam consumers, and both of them might try to scam the marketplace. That’s why an anti-fraud or trust and safety approach taken by these apps needs to be multifaceted to address the different challenges.
As an example of consumers scamming couriers, Kapoor describes a popular practice on Instacart known as “tip baiting.” In this scam, consumers would place a grocery delivery order with an unusually high tip to entice couriers. However, once the driver completed the order, the consumer would edit the tip to be much lower than initially promised. Instacart solved this problem by instituting a new policy to protect shoppers from tip baiting by requiring users to explain their reasoning for reducing a tip and penalizing users who repeatedly promised larger tips than they actually paid.
Ferraz followed up with an example of the opposite—couriers using social engineering to steal from customers.
In the scheme Ferraz describes, couriers would accept a food delivery order in the app and then cancel it. However, they would still pick up the food and deliver it to the customer’s house. Upon arrival, the driver would blame the cancellation on a glitch before pulling out a portable POS system and requesting that the customer complete their payment. Unfortunately for the customer, the POS system had been tampered with enabling the bad actor to charge the customer a much larger fee unbeknownst to them. In this case, the customer loses out on funds, and the app may lose users as trust in the app’s integrity is damaged.
How next-gen device fingerprinting can help gig apps fight back
Because many of the top gig economy apps are location-based and require users to share their location—i.e. home sharing, ride-hailing, food and grocery delivery—a device fingerprint enhanced by location technology can be a particularly effective solution for preventing fraud and policy abuses.
The end game of most gig economy scammers is not to make off with a single free meal or grocery delivery. These policy abuses and fraud scams become lucrative when they can be automated to scale. A few extra dollars in income by completing a fake delivery doesn't amount to much, but when the operation can be scaled to the size of a fraud farm with dozens of devices and multiple bad actors working together, the profits for the fraudster–and the losses for the gig app–can be significant.
Using next-generation device fingerprinting paired with spoof-resistant location technology can interrupt the scalability of a fraud or policy abuse operation by making it difficult for bad actors to access multiple accounts from the same device, or multiple accounts on different devices from the same location. However, as Andre Ferraz points out, GPS alone isn’t precise or spoof-resistant enough to be used for this purpose. Instead, the location technology has to be both spoof-proof and precise enough to identify micro-locations, such as a small apartment or room.
This blog post is a commentary on the webinar and Trust & Safety Mavericks episode #4. Listen to the full episode on your favorite podcast player:
This is an audio transcript of the Webinar: ‘Gig Economy Scams: How geolocation can help’. You can watch the full webinar here, or listen to the podcast on Apple or Spotify. Readers can also read the full webinar transcript below.
“Gig Economy Scams: How geolocation can help” transcript
Ronald Präetsch
My name is Ronald. I'm the co-founder of About-Fraud, and I'm the host of our webinar today, which is, “Scams are challenging the gig economy.” And before we go into all the details, introducing our speakers today, I would like to remind you about our housekeeping rule.
And we have only one rule, which is please be active. Please ask questions. On the right hand side of your interface, you can see a chat box. Please type in your questions anytime, we’re trying to incorporate your questions straight away in our presentation, otherwise we’ll pick them up at the end of our session. So please be active.
OK, who is here today? We have Andre and we have Vishal. Andre, please provide a short update about yourself. I think some of our audience already knows you, but please do an intro of yourself.
Andre Ferraz
Thanks, Ronald. Everybody, it's a pleasure to be here today. I'm Andre Ferraz. I'm the CEO and co-founder at Incognia. I come from a computer science background, spent most of my career as a security researcher and currently I'm working at Incognia, which is a company that specializes in location verification. And we have been doing a lot of work with gig economy companies around fraud detection and also authentication. It’s a pleasure to be here. Hope you enjoy the content for today.
Ronald Präetsch
Thank you, Andre. Vishal.
Vishal Kapoor
Thanks, Ronald. It's great to be here, great to be here with Andre. So very excited for this conversation. I am on the other side of what Andre mentioned. I am at a gig economy company. I'm a director of product at a company called Shipt, which is a grocery delivery provider. Not just groceries, other things as well. It is similar to Instacart, DoorDash, and UberEats as a delivery service. It is owned and acquired by Target.
And I am responsible for the products, I'm a director of product. I'm responsible for the products that disperse earnings to shoppers. So Shopper Earnings or Shopper Pay is a team that is under my leadership besides some other teams. As part of that, we encounter a lot of interesting scams, fraud, and regulatory challenging scenarios. This talk and the topics that we will discuss today hopefully are very near and dear to me and keep me up at night. Looking forward to an exciting conversation.
Ronald Präetsch
Then let's go from here. Perfect. Thank you for joining. Before we go into details, we have prepared some slides. I think it's good that you set the stage for the audience about some destinations. I would like to have two questions here. Maybe Vishal or Andre can give, in a short nutshell to the audience, what is actually a gig economy?
So what are criterias for the gig economy that we are really setting the stage about? What are the criteria here? And the second point is, I would like to maybe also get a common understanding, like you just mentioned, Vishal, there is fraud, there are scams, there are policies. Maybe we can also set the stage so that we have a common understanding.
Vishal Kapoor
Andre, you want to kick off with what is the gig economy, and I can take the second one?
Andre Ferraz
Absolutely. I think the quickest definition for gig economy here is basically a marketplace in which it is more, let's say, decentralized. And there are service providers on one end, there's usually the consumer on the other. And the service provider is independent. They're a gig worker and they're doing this for a living or to complement their income.
And with that, it brings another level of complexity. When we compare this to ecommerce, ecommerce is more centralized and also the goods that are usually bought on ecommerce platforms usually take longer to be delivered to you as a consumer. When we're talking about gig economy apps, usually the consumer is expecting something immediately. When they're shopping for groceries online or they're requesting a ride on a ride hailing app, or when they're ordering food online, they expect that to come very quickly. This brings another level of complexity because these companies cannot wait and manually verify those transactions as it occurs traditionally in ecommerce.
Ronald Präetsch
I would, at least from my point of view, maybe add two keywords here. One is it's complex and it's on demand in many cases. And I think that's the beauty when we talk about the fraud challenges or abuses, et cetera, for this gig economy, because it's complex, it's challenging, it's dynamic, it’s changing, a lot of parties involved, but that’s the exciting part.
Vishal Kapoor
I can take the second question that you were referring to, Ronald. So what is exactly a fraud versus, for example, a policy abuse? How do we define it? How do we categorize it as one problem versus the other? If you think about fraud, fraud is—the way you classically or the companies classically think about fraud is your typical, it's almost something illegal that somebody is doing. You're not supposed to do that. It's your typical stealing identity or taking over somebody's account or switching bank account so that you can actually get money deposited into your account. Things like that in a black and white sense fall in the category of fraud.
If you think about policy abuse, what is policy, what is abuse of policy, and what kind of scams are there? That is where it becomes a little bit more gray. I'll give you a simple example, and this is a made up example. This isn’t how we work.
But just as an example, suppose that you have a delivery service, let's say, for example, a service like Shipt, which has a policy where they are giving the drivers or the shoppers who are making the delivery, the independent contractors, they're incentivizing them to return an undelivered order. So let's say they try to go and deliver an order to a customer and for some reason they could not find the address or the customer was in an apartment complex and they couldn't access the customer.
There could be many reasons, but in order for them not to just trash that order and walk away, maybe the company, such as Shipt, will incentivize them. “Will incentivize” meaning “will pay them to actually come and return that order back to store.”
So let's take a very simple example here. Let's say that we pay $5 or Shipt pays $5 for an undelivered order to be returned back to the store. Now, there are situations. The way companies work, many of these companies, the way they work is on a principle of unit economics, which is per transaction, per unit of job, per order, per service, per booking, for example, for AirBnB.
If you think about that, what is the cost per unit of your foundational business model or your transaction model? In this case, let's say that the way the business is working is that the unit economics for delivering an order, let's say that that particular driver is delivering, let's say, twenty orders and every order is supposed to give them $4. The unit economics is $4.
Therefore, for the entire batch of orders they make $80, which is a significant amount. Now, the question about the policy is when it comes to the last order, especially when it comes to the 19th or the 20th order, should the shopper actually try to deliver it and get $4 or just return it and get $5? This is where there is a policy. If the policy does not really work with the unit economics model, then it can lead to policy abuse.
This is one example, a simple example where I'm using a monetary policy. For example, there are other policies, there are product policies. Where do you allow, where do you add friction? How much do you want to oversee somebody? How much do you want to regulate somebody at the risk of using that word, in order to maintain the quality and the purity in your platform? Meaning if somebody comes and says they are the ones who are doing the job, are they really the ones who are coming and doing the job? Or did they switch with somebody else?
There are many things like that which are non-monetary. That could be another policy. You could have some checks and balances in place to do some verification in the moment.
For example, there are various things in the funnel when it comes to fulfillment. Many, many areas where you can have these different policies, and sometimes, as I said, it lends to policy abuse. Sometimes the people who signed up to do the job are not the people who are actually doing the job. Sometimes a monetary policy may incentivize them to, instead of finishing the job, return it and make an extra dollar in the example that I gave you and so on and so forth.
That is the distinction. Fraud is really, really black and white, which is you're supposed to do, you're doing something really illegal, you're taking over somebody's account, you're switching bank accounts, you're scamming somebody out of the money, or something like that. Policy abuse is a little bit more if that answers the question.
Ronald Präetsch
Thank you for this detailed explanation, but I think everybody can see there's a bit of overlap between different topics. That's why I'm very curious now to get from Andre his perspective about this complexity and what's really happening behind the scenes. So Andre, maybe you can also provide some basic information here.
What's the topic right now? A new term, trust and safety. I think many of us are very familiar with trust and safety, but maybe you can also spend a few sentences talking about what it is and then going through all the details.
Andre Ferraz
Excellent. This part of this presentation is to share some of these complexities when it comes to gig economy apps. Then the broader term trust and safety is, let's say, a superset of fraud that encompasses scams, policy abuse, content moderation, and other things that occur on online platforms. It's a broader definition to state how these platforms are ensuring that their customers can first of all, trust the platform and be safe being part of it when it comes to the gig economy.
More specifically, the four things that are brought here to help you understand why this is a more complex space is, number one, it is more fast paced. Like I mentioned at the beginning, when we're talking about ecommerce, we usually wait a day or two to receive your order. The platform usually has more time to verify. For example, when a suspicious transaction occurs, you cannot do that in the gig economy space, right?
Let's imagine the scenario in which someone is ordering food online. That transaction cannot be frozen because otherwise your food is getting cold. That needs to happen at a much faster pace. This creates an additional complexity when dealing with fraud and policy of use. The second thing here is that there are more actors involved in the process. Usually when we're shopping online in traditional ecommerce, you as a consumer are interacting with the ecommerce company. If it's a marketplace, ecommerce would abstract that for you and would manage shipping and payments, et cetera. You don't even have any interaction with whoever is providing you with those products.
And in many cases, the ecommerce website also has their own products that they deliver to you. It's a one to one relationship. When it comes to the gig economy, you usually have the store or the restaurant, you have the consumer, and then you have the gig worker. Basically, you have another party here involved that makes it more complex.
Now the combination of attacks here can happen in any way. You have cases of consumers scamming the drivers, drivers scamming the consumers, fake listings, fake restaurants, for example, or fake stores. You have all of these types of things going on, so it's more complex.
Third thing is that these actors are able to communicate directly, so this brings another level of complexity. For example, the driver or the gig worker, they can interact with you as a consumer. They can send you a message, they can call you, and the opposite is also true. As a customer, you can interact with that gig worker. Once you connect to people online, anything could happen. Any type of social engineering scam can occur in this environment.
And then finally, the fourth point is that, in most cases when we're talking about the gig economy, we're talking about local services. Grocery delivery, food delivery, ride hailing, for example, all of those things have a local component. Location services inherent to itm—most of these apps would rely on location services to deliver their product or service.
It is quite easy for bad actors to spoof location information. Unfortunately, or fortunately, the operating systems, both Android and iOS, for example, have built a feature for developers so they could test their product, their application, as if they were in a different place. Let's say I work in a global app like Facebook, for example, and I have released or built a feature for a specific market. Let's say I built a specific feature for the UK. How would I test this if I'm in San Francisco? I need a way to test this feature. Then the operating system is enabled—when the user is on developer mode—to change their GPS coordinates.
But unfortunately, this is exploited by bad actors to spoof GPS information. You've got to make sure that you can rely on the location data. Otherwise this could trigger other types of fraud and policy abuse So these four things here make the work of fighting fraud and policy abuse in this space a lot more complex.
Kudos to you, Vishal, I know how challenging your job is, but those are the points that I wanted to share. Probably you have something else to add as well.
Vishal Kapoor
Yeah, I would love to add to a couple of things that you [mentioned], especially in bucket number two, where you said there are many actors involved, like customers, drivers, restaurants, or shoppers or drivers and restaurants.
You gave an example of location spoofing or location manipulating, essentially, because you want to use it for a legitimate case where you want to test, you want to emulate a user, which is actually potentially somewhere else. That's not really abuse, but that feature can lend itself to abuse. This is a classic example of a knife that you can use to cut vegetables as well as you can use it to do some harm to somebody else. It's always a two-edged sword, for example.
I'll give two other examples, hopefully to take the conversation forward. One of them you touched upon: this is customers scamming drivers or customers scamming shoppers, and I'll give you an example of how that happens. It's very interesting. The other thing is drivers actually scamming the marketplace, if you will.
Let me give you the first one, customers scamming shoppers.
There is a feature in Instacart, which is one of our competitors, where what they do is what customers can do. This feature has evolved over time, but what customers were able to do was they would add a tip as part of placing an order. And what Instacart was doing was to make the order enticing for a shopper or for a driver to pick up and go to the restaurant. They would actually show what tip is part of that order as well. Ultimately gig workers are coming to the platform for a parallel income. They want to make a living. Obviously, you want to be upfront about all the bonuses as much as you can without confusing them too much. You want to be upfront about all the bonuses, all the tips, all the extra income that they will make on every order. If somebody is generous and somebody is willing to tip 30%, 40% on an order, it makes sense for the platform because the platform is just an intermediate thing.
It makes sense for the marketplace to actually transfer that knowledge instead of hiding it. Transfer that knowledge to the supply side, the drivers and the shoppers, so that they can make a more informed decision if they want to fulfill that order versus other orders. In this case, customers started doing something called tip baiting, which is they would add a high tip and that would motivate shoppers to take the order and post delivery. There was another feature in Instacart post-delivery, they would allow them to refine their tips because tips are really based on the service that you provide somebody. And the customer was very much empowered to say, “I did not like the service,” at the end of it. “My food was cold, so I want to reduce my tip.”
This became a pattern where customers would put hundreds of dollars of tip in order to entice the shoppers and drivers to pick up their orders versus everybody else that they were seeing and then at the end of it, when the order was delivered, they would go and reduce the tip. So this is customers, let's use the word customers, scamming the drivers. That can happen, which is very unusual, you wouldn't think of this happening on these two sides.
What Instacart did as a result of that—there was some regulatory pressure, because we have to be mindful that, especially when it comes to the gig economy, a lot of this is sort of scrutinized. A lot of this is watched very closely because these are independent contractors. A big push in the industry and push generally in the public policy space is to be fair to these people, not be exploitative. Pay them fairly, pay them well. Especially the people who were trying to do a good job. There was some regulatory pressure from lawmakers and things like that, due to which Instacart then implemented a new feature, a new change in their policy, which was if a customer is reducing tip post delivery, then they have to actually provide a reason why they are reducing the tip.
And if that happens multiple times, then it's like you are probably baiting tips, so you're probably abusing a policy.
These kinds of things happen on both sides. There is another example about shoppers trying to scam the company. We can come back to it later, but that was one example that came to mind to take your idea forward.
Ronald Präetsch
Thanks for your, let’s say, tangible example. I think it's always good to really connect this to some slides because again, that's why we are here, really sharing the insights, sharing good examples, giving some ideas to the audience as to what you might look at in protecting the business or the customers.
It's complex, but now going from the complexity into, “What are normally the use cases?” or “What are the typical cases?” We already touched on different cases but as you can see here, it's quite a big range of fraud types or abuse types which are happening because, at the end of the day, a fraudster wants to make money.
And that's also one point, Andre, if you're going through maybe we can also look at what is the exit for fraudsters? Stealing one meal is normally not the goal, but often they want to make cash somehow. Maybe when you go through, maybe you can provide this kind of chain. How is it getting the cash out of the system?
Andre Ferraz
Here we have a lot of examples of different types of policy abuse that occur in gig economy apps. I won't go through the whole list, because there are a lot of different things here. Maybe we should switch to Vishal because he has the hands-on experience to share a few of the things he's seen, like the one he just described with Instacart, which was quite interesting. Vishal, any thoughts here on the most common things you've seen?
Vishal Kapoor
Yeah, I think the most common thing that we worry about, that keeps us up at night is definitely everything, at the end of the day. As Ronald mentioned, it leads to them trying to extract money out of the platform. Policies, which is the example that I gave before, about the $4 versus $5, $5 versus $4, fiduciary policies are very likely to get abused right at the top. Fiduciary policies or even fiduciary infrastructure, fiduciary systems. What do I mean by that? Bank accounts, like account takeovers. The way Doordash, Instacart, the way these different companies work is—well, at least Instacart and Shipt, Doordash is a different model. But Instacart and Shipt, the way they work is they give you a physical debit card, which, imagine yourself going and doing the groceries. A shopper is no different from you. They are just doing the job on your behalf. When you do the groceries, what you are doing is you're going to the store, you're going around the store, you're basically picking up items, putting them in your cart. You're actually going to the checkout counter, checkout line. You're actually swiping your car and then you're walking out, loading the items in your car, you're driving around and then you're unloading. Wherever you live, it's the same experience foundationally, except somebody else is doing it for you. The card aspect is the fiduciary, the financial thing that I was talking about.
Instacart and Shipt, for example, give debit cards, physical cards or even virtual cards to the shoppers. And what happens is, as soon as the shopper accepts an order, let's say that I'm a shopper and I accept an order, and that order is supposed to pay me $20. After I finish and deliver that order, the card will actually have that it is going to pay me $20.
But the order itself is a $100 order, because the customer is paying $100 to get that order delivered. So the card would be loaded with $100. Because when I go to the store, I want to swipe that card. When I do the shopping, I want to go and swipe that card and then do the delivery and so on. This is not an insurance claim that I'm putting my own money and then submitting a claim back to the company. It happens in real time, like you said. Those things like card abuse, card takeovers, somebody else impersonating somebody else's identity, taking that over, etcetera. Those things are likely to get abused the most. Policy abuse, obviously, as product policy abuse, when we see that, when we see that there are certain things at the bottom line, which are causing an impact on the top line.
Generally what happens is you will see some part of product or something, $1, $2 here or there, which is actually causing a high level impact on the top line of the business. The finance team will tell you, your legal team will tell you, somebody will tell you those things. It's whack a mole. You see that you add some friction, so that you plug that, and then somebody else comes up with another creative idea of doing something. But generally, the abuse mostly happens where people try to switch bank accounts, people try to switch other identities, people try to steal other people's debit cards. They will call customer service and impersonate somebody and say I am that shopper, when they're really not.
This is some person sitting somewhere in Russia, for example, right? But they are impersonating somebody and trying to do everything online.
I'll bring one more example, which is the gig worker app fraud, which is again sort of “Where is policy?” and “Where is fraud?” It's a little bit crazy, I'll say that, and this might help the audience understand a little bit more. Again, let's come back to the scenario where you and I go to shop and buy our groceries, right? We go to a grocery store, we are trying to buy milk, we generally buy 2% milk. But in that particular time the store is out of 2%, and we find 1%, and we take 1% and we walk away. That's fine for a shopper when they are fulfilling the job. For somebody else, there are one of two things that they can do or one of three things that they can do.
Maybe the customer has already expressed a preference that if you don't find 2%, get me 1%, so the shopper knows what to do. They will just pick up 1%. The second option is they can try and talk to the customer. If there is no preference, they will try and talk to the customer.
The third thing is, suppose there is no milk at all. They still want to complete the rest of the order. They will go and say there's something out of stock. They just mark that item as out of stock and move on. That is a policy that is allowed. There are genuinely items, there are genuinely things that may not be available in the grocery store. A lot of this actually falls into—just to take a side step into how we build technology—is anticipating the inventory levels inside a store, how fast they are depleting. We have machine learning algorithms that actually monitor the catalog and try to figure out how fast these things are getting depleted. Because if we are taking an order with ten items from a customer and nine out of those ten are not available, it's a very bad experience for the customer. If they are spending that money and all they are getting delivered is just one item out of ten, that's not a good experience. We shouldn't have taken the order in the first place. There are forecasting systems, prediction systems that try to figure this out.
But coming back to it, a shopper is allowed to say that this was out of stock. Now, what happens is when there are bad actors, they will go to the store. Sometimes they won't, even—as you said, they can spoof location. They can get into a store and say everything was out of stock. Now, the policy is that if you are trying to do a good job, our policies that we always—and all platforms, as I said, part of this goes into how we get regulated by lawmakers. Part of it is that we have to pay fairly. If somebody has done a decent attempt and, let's say, a genuine case where somebody actually went to the store and items were not available, we are liable to pay that independent contractor, that gig worker. We are liable to pay them because it's the right thing to do. They made the effort so we should pay them.
But then there are bad actors who pretend that they are at the store and they can mark everything out of stock because the product allows it. Now what do we pay them? Do we not pay them? That is where the other example, which I was referring to before, where this gig was fraud, can happen. There are many, many such examples that can happen. This is one area and then again we go back to repeat offenders like how many times are they actually doing it? Are they doing it over and over again? If they are doing it and some other shopper comes and another shopper is not doing it as much as this one shopper is doing it, then do they have good intent or do they not have good intent, inferring some of that?
There is an operations team at the company as well. We look at individual scenarios, we look at individual data points and shopper behavior and all of that. There are these kinds of examples which happen in the real world.
Andre Ferraz
Yeah, this is incredible. There's another one that I wanted to share that was quite impressive to me. Very smart social engineering scam where as soon as the gig workers got the order, they would cancel it. They have the ability to cancel the order, but they would actually show up at your house, they would bring the food, they would bring the receipt, and then what they would say was, sorry, there was a bug on the app. You received the notification that the order was canceled, your payment didn't go through. And then they would have a portable POS system right there and they would say, you can pay it right now. Here's everything, everything is good. But then what was tricky here was the portable POS machine was actually tampered. So when they typed like $50, they were actually typing $5,000. You would swipe the card, the transaction would be settled, the person would get in their car and drive away and you just lost $5,000 because of that scam. So a very lucrative scam for the gig worker.
That was quite challenging because again, the communication was actually happening outside the platform. The person showed up at your house, the platform wasn't able to see that happening. Really tricky for the platforms to fight all these things that can happen. It's a much more complex environment for sure.
Vishal Kapoor
Yeah, and like I said, the example I gave was the supply side or the drivers or shoppers scamming the actual platform. What you are saying is them scamming the customers. So now we have seen things on all three sides. Customers actually scamming the drivers, the drivers scamming the customers as well as somewhere in between. That happens.
Andre Ferraz
Yeah, exactly. There was another one I've seen. I think it was also in the grocery delivery space. What would happen was that with this delivery service, they had a fintech product as well, in which the gig workers could create a bank account with them. What happened was the consumer, in this case, it was the consumer attacking the gig worker. The consumer would get the contact details from the driver and they would call the driver as if they were from customer service and they would say, “Oh, someone is trying to take over your bank account here with us. We need your help to fight this issue.”
At the end of the day, they would say, “I'm going to send you a code to your phone number. Please tell me that code so I can start the process here to secure your account.” That was basically the credential for the fraudster to commit account takeover. Then the gig worker, they had money on that account. Once the account was taken over, they would get the money and the gig worker would lose it all. All sides are attacking each other here. It's a pretty complex space.
Ronald Präetsch
The question is now, how can you actually detect this? Of course, if you have a repeating pattern and someone is doing this all the time and customers are complaining, you might get it. But I also assume from my point, like device fingerprints, certain transaction data might be not so helpful anymore, so that's why location data could be an interesting angle where you actually have a different perspective on what's really happening.
Maybe Andre can provide some perspective here from your project or experience on how location data really makes a difference and is it the golden bullet, location data? Or how is the game changing with having this different level of information?
Andre Ferraz
One important thing I have to say before I get into the specific techniques to fight this type of fraud is that reaching 0% fraud is almost impossible. I won't say it's impossible because sometimes it can happen for a short period of time, but it can happen. What you really need to do is to prevent fraud or policy abuse from scaling. You need to, as Ronald just said, identify the repeat activity. You need to identify those patterns and you need to block those things specifically so you prevent it from scaling. I'm going to pass it to the next slide here for some additional data about how we are working with gig economy apps to find this type of thing, which is that the fraudster or bad actor in general, can hide behind multiple identities.
It's very easy for someone to create, for example, a fake email address or to get a burner phone number, or even to create a fake document. This is very accessible for fraudsters and scammers, they know how to do it, and they will always find ways to create new identities to perpetrate this type of fraud. They also sometimes, depending on how lucrative the fraud scheme is, they also may have access to a big number of devices. They can switch devices, they can even buy new phones. They can even operate as a team.
And we see this quite frequently actually, fraudsters getting together to operate as an organization, not only an individual. What you really need to do is to try to find a link between these identities, between these devices. And so far, given that location is so central to these types of applications, we have identified that location is a good way to find that link between these multiple identities. The most basic thing you need to do here is really to have a strong device ID or a strong device fingerprint. You make sure that, for example, a single device cannot open multiple accounts, a single device cannot access multiple accounts. By doing that, you will be able to address a significant number of the cases, but not everything.
Then to prevent it from scaling. The challenge here is, okay, in case this fraudster or bad actor has access to multiple devices, how do you link them together? And then this is where location comes in. If we see that all of the devices always come back to the same location, this is probably the same person, right? So how do you do that if spoofing GPS information is so easy? First of all, you need to find ways to detect location spoofing. There are multiple ways of doing that, including for example, identifying if that device has any GPS spoofing app installed.
If you search on the App Store or Google Play right now for “fake GPS,” for example, or “GPS spoofing,” you're going to find hundreds of apps that enable you to do that. Simply analyzing if the device has one of these apps, you can understand if there is a risk of GPS spoofing from that device. That's the first and most basic layer when it comes to location. The second thing is to identify misconfigurations or security vulnerabilities on that device. There are a lot of things happening, for example, around tampering with the application. There are some apps that enable you to even change the source code or to intercept calls to the back end and manipulate those things, manipulating the data that is sent to the server and things like that.
You have to make sure that you are able to detect these things. Detecting routing, jailbroken devices, emulators app tampering, app cloners. You have to do this kind of thing to identify this type of risk. And this is a way to also flag the risk of location spoofing.
And then finally, the last piece here is that you need to have something else to locate that device, not only the GPS, right? Because if spoofing GPS data is so easy, how would you identify that? In our case in particular, we analyze all your sensors like WiFi and Bluetooth for example. And by doing that, we're able to see if there is a mismatch between the WiFi based and Bluetooth based geolocation through the GPS coordinates that the operating system is sending to us. By finding these mismatches, we would say, “Okay, this is a user spoofing GPS information.”
Those are the three steps to detect location spoofing. There's a lot more, but I won't spend a lot of time here on this. Once you have good location spoofing detection capabilities, then you can start relying on the location data. The last piece here is the precision of the location data itself. Let's say that the fraudster operates from an apartment complex. If you are relying on GPS data, unfortunately you cannot use that data to block users. Why? Because the neighbors of that fraudster have nothing to do with it. You cannot block those people, and GPS data only allows you to have an understanding of where this user is in terms of the building or the block in which they are located. If you want to use location data to block bad actors, you have to make sure that you're super precise and you are identifying the specific apartment in which the fraudster operates.
We work with this concept that we call fraud farms. Identifying a fraud farm is basically the idea of identifying a single apartment or single home, for example, from which we identify a lot of suspicious activity. Let's say that there is someone creating multiple accounts or accessing multiple accounts from the same location using many devices. This is not normal activity. There's no huge family with ten people that suddenly have the same idea of creating an account on a food delivery app or grocery delivery app, right? If you find that type of activity, you can block that specific department and you're going to make the life of the bad actor much harder because they will now need to not only switch identities, switch devices, but now they have to start switching locations and that becomes super expensive for them. They would probably go somewhere else to attack another platform instead of insisting on doing that, because it's not that easy to rent a new apartment to continue your fraudulent activity. They would probably start attacking a different app.
Ronald Präetsch
The interesting part is fraud fighting is always at different levels and reacting right now, providing content at different levels.
Thanks to Vishal for being active on the chat and providing feedback. But I would like to take one question, which I think is relevant for scaling, for being a global player. It's about privacy. Andre, you mentioned you can collect a lot of data about someone connecting the data points, really looking at behavior. One question to you Andre, how do you make sure you're really compliant to, let's say, local rules?
Then the question to Vishal is, I assume a lot of gig workers know that they are tracked everywhere. Do you believe they are scared about this or they're completely okay? Or maybe see it as an important factor from the trust and safety side that you as a marketplace can actually track the behavior. That’s an interesting perspective. It's always easy to talk about data and technology, but often leave the human behind. I think that's also an important point to always consider.
Absolutely. This is super important, especially now where we have all these new regulations around privacy and data protection. I would say that the two most important things here are one, making sure that you only collect the data, in case of location data, for example, after the user consents to it. When they download the app and they say, “Okay, I'm okay sharing my location information with this app.” Once the user says yes, you can collect the data.
There were some cases of companies in the past that tried to collect this type of data without the user's authorization. It's not going to play well. This could actually become a scandal for the company. First of all, if you're collecting this type of data, make sure that you are asking for permission. The second thing is that usually when we're talking about behavioral information, and that includes location data, ideally you're not mixing that with the PII information. You're not mixing the location behavioral history to the user's name, phone number, email address, things like that, that could identify the user in our society.
Why is this important? In case this type of data is leaked and people get access to it, if they're not able to identify who's the individual, the risk is much smaller. Making sure that these data points are in different silos and you have very strong security to protect this type of data is important. That's the approach we take as a company.
Incognia does not ingest any PII data other than the location and device information. Who keeps the PII data is the platform, is the app. We only take care of the device and location information. We basically have a wall between these two parts here. The platform, which is who really has the direct relationship with the consumer, holds their personal data. We analyze the behavioral and device information. For us, the end user doesn't have a name, doesn't have a phone number, doesn't have an email address.
Vishal Kapoor
I can provide a slightly different perspective on the same lines, Andre, than what you just mentioned. Before Shipt, I was at Lyft, which is the other big ride sharing company in the United States. And exactly to your point, there was a concern that, at least being a ride sharing company, we would track, we would get the location where the request was coming from.
Just two simple points that we had to track were where the request was, where we had to pick up somebody, and where we had to drop them off. Those two were necessary. Getting the locations for those two points was always necessary in order to fulfill that request.
Getting the latitude and longitude for those two, you can imagine, because Lyft being a company which also knows about the customer as well as has his data of where the origin and the destination data for the customers, for example, there were concerns.
There would be concerns where, what if one of those lat-longs was actually, for example, an Alcoholics Anonymous location? Or what if it was a therapy institution, for example, or a therapist? You don't want to cross over into what that person is trying to do. Location tracking can lend itself to doing that.
But coming back to what you said, Ronald, I think the way large organizations who have this [problem] work around it in two ways. One is they try to have something similar to financial data.
If you think about financial data, analytics, like company level analytics forecasts, especially public companies which report to the street, there are people who have access to forecast, to information, etc. that may move the stock price. And such people are actually protected by blackouts, blackout windows. I'm just giving you an example. They are not allowed to trade shares a month before and a month after the earnings, after they declare the earnings. For this reason, because they have more information than the general public in order to maybe sell shares or buy shares if they think the performance will be higher or lower. There is a special category of people, a class of people with special rules who have access to that data. That is one thing that companies do.
The second thing is external lawmakers. There are policies like CCPA or GDPR where we give full control to the users to control their data. This was a big thing, I believe, in 2018, 2019 when GDPR was actually implemented, was allowing users, giving them the guarantee that if they want, if they initiate an action to delete, to remove themselves from our systems, initiate an action to delete themselves, we are legally bound to actually delete their identity completely.
Those are the two ways people who have access to that data are special. There are people that need access to that data sometimes in order to build products, make decisions, et cetera. And then beyond that, now there is the law, the law of the land is that if somebody wants to delete themselves, erase themselves from something, then companies are responsible and accountable to actually erase them from the systems. Those two, beyond the fact that we do collect that data, there are certain measures in place that the companies use to actually alleviate some of these concerns for the users.
Absolutely. There's something else I wanted to comment on here, which is another layer around this, the operating systems themselves. You have the regulators, you have the operating systems, which sometimes are even more powerful in these types of scenarios. And there are two things that they have a very strict policy on, which is around device fingerprinting and location data. When it comes to these two, you have to disclose to the operating system, to the platform, the reason why you are collecting this type of data. If you're collecting this type of data to provide your service, to secure your users, to prevent fraud, this is all fine.
They do have exceptions for these use cases and they allow the apps to collect for these purposes. For example, if you want to use this data for advertising purposes, you're probably going to be blocked. Apple and Google, they would say you cannot use device fingerprinting and location data for this. We're seeing the impact of this in the ad tech industry.
For example, the app tracking framework that Apple has released is now blocking the ad tech vendors from using ways to uniquely identify a device. For that use case, you cannot do it, but if you're using it for fraud prevention purposes, security purposes, you can do that.
Ronald Präetsch
I would like to, in the last few minutes, touch slightly on a topic which is related to many things we discuss. We talk about machine learning and also, which I mentioned, is in one of the messages, you need to provide feedback. And if you have now fraud, abuse and other types of strange behavior, would it make a difference, Vishal, to flag certain transactions or certain behaviors with different flags? Or would you have different machine learning models for different appuit types?
So having a machine learning model for the gig worker, one for the customer, one for the shop, or is it somehow related to one model and how would you actually give feedback?
We know feedback—sometimes, manually, someone's looking at this, and otherwise maybe it's detecting feedback from an external system that gives a score and then you take the score to train machine learning models. How are you making sure this kind of different type of fraud is really tracked in the right way to make the right conclusions for the machine learning model?
It's sort of a chicken and egg question—do you start with humans? At what point do you actually move it to AI, like machine learning and artificial intelligence? I like to say—I think Andre kind of covered this in spirit, I don't think he said it—but I like to always call it human artificial intelligence. Which is, it is always a combination, even with the models, if you think about it. There is a risk that the models sometimes, a lot of times, need other supervision because they might train themselves and they might go out of date because the world is changing, the models may not train fast enough, they may predict something weird, etc.
For example, there is a form of abuse prevention in Lyft and Uber. I'll give you a very simple example. When there is a route, when you take a ride, as a part of trust and safety, the car is supposed to drive on that route, which is actually specified by the maps by Lyft. If you deviate from that, right, if you stop, if you take a stop, and you stop somewhere, you know, pull up on the side of the road or take a side highway or something like that, that actually can trigger a red flag because it could potentially be a safety issue for that customer.
And the companies take this very seriously, not so much in the US. But you know, we have to remember that Uber, for example, operates internationally. Countries like Brazil, India, et cetera. They want to be very careful about their brand, image, safety and so on and so forth. So yes, when there are millions, billions of rides happening on the platform, you cannot really have humans monitor every ride that goes take a side road or everything that is happening.
You have to automate a lot of that. But really the problem that we were talking about before, some of the shoppers scamming returning more than normal, things like that. Typically what happens is that you start with a machine learning model that is trying to run the product. Then what you do is typically you have some sort of external system which is an anomaly detection, some sort of anomalies, which is like all these shoppers, do they have aberrant behavior? Are they behaving like normal shoppers? Do they take a lot longer? Do they take 5 hours to deliver an order whereas everybody else takes 30 minutes? Like that could be something to flag upon, right? So there are many things like that which are rule based, which people generally people develop.
You know, it's easy to develop a rule based system and look at the database and these timestamps, et cetera, which you're tracking. It starts there and then somebody will generally write a script or write some sort of a simple program which will generate some alerts and notify the operations teams. And the operations teams will usually the human intelligence, they will usually fire some warning shots. They will look at certain people. If they are actually misbehaving, they would go and do some human review. One of the questions that came up was what if somebody behaves well in the past and now has started misbehaving? They will look at the history. It's not a machine learning model. They might just go and block a certain shopper, but that's maybe not the right thing to do because it is circumstantial. At the end of the day, we are working with humans who are imperfect, right? Other humans in operations teams, for example, might look at that, might fire some warning shots, ask them, communicate with them, did something go wrong?
Why did you take 5 hours to deliver an order which we thought it would only take you 20 or 30 minutes to deliver? Those things happen. But beyond that, if there are things that happen at scale, like I said, people deviating from a route, taking side lanes and all that, then you have to build a machine learning model where you're trying to figure out if, depending on the context, who was the passenger? Was it a female, a young female in the car? What country was this?
Is this in an urban area or a suburban area? What was the time of the day? There are so many factors which a human cannot actually objectively evaluate. You need machine learning systems to flag that. And again, some of these problems need immediate flagging, immediate reactions. In this case, for example, the Uber safety team will actually directly call the customer, the rider in the car, and try to initiate a call and ask them if you feel safe or send them a notification saying, do you feel safe? Did you intend to do this? Or you feel like and if they don't respond in a certain time, then there are other measures that the team has to take. There's a protocol. If they don't respond in time, that means that could be a security incident, for example.
It starts simple, but it depends on number one, it depends on the scale of the problem and what you're trying to do, how fast you have to react, like Andre mentioned. Even in the on-demand economy, although these are faster use cases compared to ecommerce, even, there are cases which you have to react instantly, whereas there are cases that you may react in an hour or 15 minutes or at the end of it, or you can stop the bleeding. Like, fine, you lost money today, but maybe you can go and stop the bleeding tomorrow. Right? That is acceptable.
Versus if somebody's security and their wellbeing is at risk, you actually have to react right now. The second case needs machine learning. The first case can probably use a combination of human versus some sort of, like, low level coding versus building a full blown, expensive machine learning system.
Ronald Präetsch
I feel we could talk about this for hours.
I mean, that's the beautiful thing about this gig economy, that there are so many different areas and types and players involved, which makes it really interesting. But we're already reaching the hour, and I would really like to respect everybody's time for today. So that's why I would really like to say a big thank you to Vishal for joining our session today. Also to Andre, thanks a lot for sharing all these insights from your projects and certain insights how technology can help, let's say, the marketplaces to identify and also to protect customers, the business model, and also the whole economy. To the audience also, thanks a lot for joining today. If you have questions, please let us know. You also get a recording afterwards. Yeah, thanks a lot, and stay safe, and see you next time. Bye bye.
Vishal Kapoor
Thank you, guys. This was a great conversation. I really enjoyed it. Thanks.
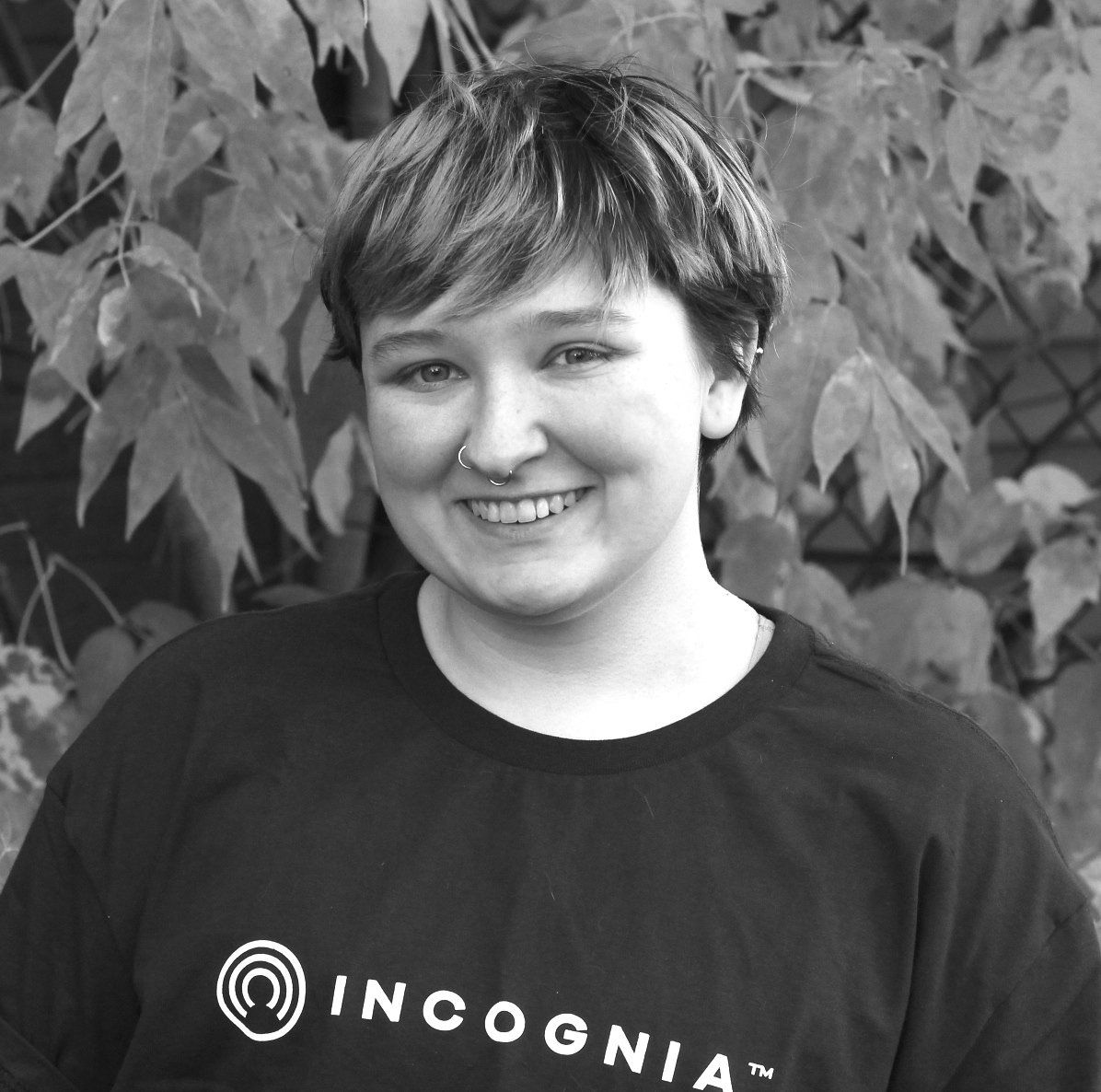